Apache Spark Training
Apache Spark training provides in-depth knowledge and hands-on experience in using Spark for processing large datasets in a distributed computing environment. Whether you are a beginner or have some experience, Spark training can help you master data processing, real-time analytics, machine learning, and more.
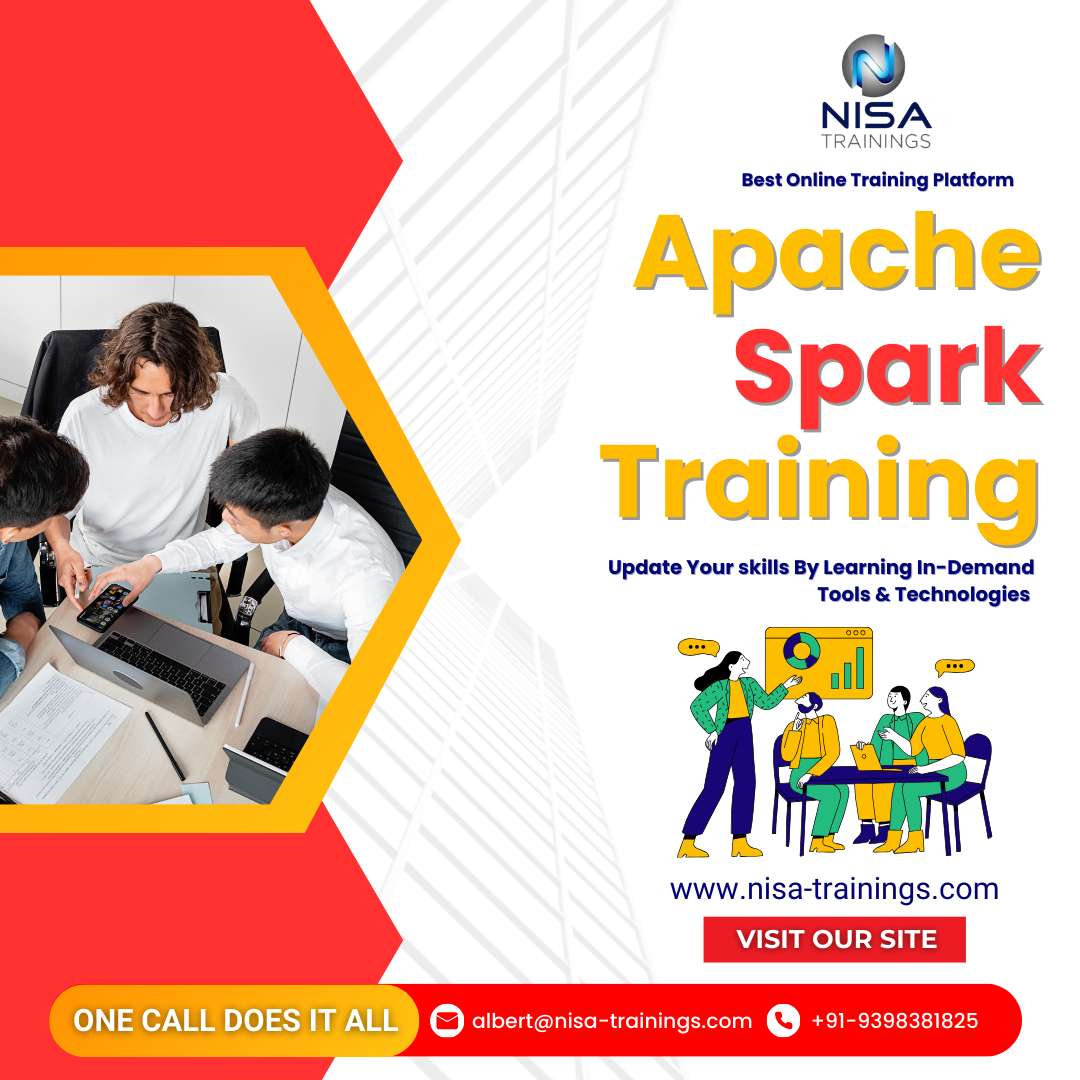
Why should you choose Nisa For Apache Spark Training?
Nisa Trainings is the best online training platform for conducting one-on-one interactive live sessions with a 1:1 student-teacher ratio. You can gain hands-on experience by working on near-real-time projects under the guidance of our experienced faculty. We support you even after the completion of the course and happy to clarify your doubts anytime. Our teaching style at Nisa Trainings is entirely hands-on. You’ll have access to our desktop screen and will be actively conducting hands-on labs on your desktop.
Job Assistance
If you face any problem while working on Apache Spark Course, then Nisa Trainings is simply a Call/Text/Email away to assist you. We offer Online Job Support for professionals to assist them and to solve their problems in real-time.
The Process we follow for our Online Job Support Service:
- We receive your inquiry for Online Job
- We will arrange a telephone call with our consultant to grasp your complete requirement and the tools you’re
- If our consultant is 100% confident in taking up your requirement and when you are also comfortable with our consultant, we will only agree to provide service. And then you have to make the payment to get the service from
- We will fix the timing for Online Job Support as mutually agreed by you and our consultant.
Course Information
Apache Spark Training
Duration: 25 Hours
Timings: Weekdays (1-2 Hours per day) [OR] Weekends (2-3 Hours per day)
Training Method: Instructor Led Online One-on-One Live Interactive
Sessions.
COURSE CONTENT :
1. Introduction to Big Data and Apache Spark
- Overview of Big Data: Understanding the concept of big data, challenges associated with processing large datasets, and the need for distributed systems.
- What is Apache Spark?: A quick introduction to Spark’s architecture, components, and why it is faster and more flexible than other big data frameworks like Hadoop.
2. Setting Up Apache Spark
- Installing Spark: Setting up Apache Spark on various environments (local, cluster, cloud).
- Configuration and cluster setup: Using Spark in standalone mode, on Hadoop YARN, or using Mesos.
3. Spark Programming Model
- Resilient Distributed Datasets (RDDs): Understanding RDDs, the core abstraction in Spark, including their operations and transformations.
- DataFrames and Datasets: Introduction to higher-level APIs in Spark for structured data processing, including SQL support.
- Operations in Spark: Actions vs. transformations, lazy evaluation, and fault tolerance.
4. Spark SQL and DataFrames
- Using Spark SQL: Querying data with Spark SQL, working with DataFrames, and applying SQL queries on structured data.
- Data Sources: Loading data from various data sources such as HDFS, S3, Parquet, Avro, JSON, and relational databases.
- Optimizing Queries: Understanding Catalyst optimization and Tungsten execution engine for performance improvements.
5. Advanced Spark Concepts
- Spark Streaming: Real-time data processing with Spark Streaming using DStreams and structured streaming.
- Machine Learning with MLlib: Building machine learning models using Spark’s MLlib library.
- Graph Processing with GraphX: Working with graph data using Spark’s GraphX library for graph analytics.
6. Spark Performance Tuning
- Caching and Persistence: Optimizing memory usage in Spark applications by persisting data.
- Shuffling and Partitioning: Improving performance by controlling data movement and partitioning strategies.
- Understanding Spark UI: Monitoring and debugging Spark jobs using the Spark Web UI for job optimization.
7. Spark Deployment
- Running Spark on Different Clusters: Deployment on Hadoop, Mesos, and Kubernetes for scalable distributed processing.
- Working with Cloud Services: Using Spark with cloud platforms like AWS, Azure, or Google Cloud.
- Job Scheduling and Monitoring: Integrating with cluster managers, scheduling jobs, and ensuring reliability with job monitoring.